Jill Mesirov Laboratory
Research in the Mesirov lab focuses on applying machine-learning and statistical methods to functional genomics data with the goal of better understanding the underlying biological mechanisms of disease, improving stratification of patients with respect to different treatment outcomes, and identifying novel drug targets. Our lab is committed to the development of practical, accessible, open source software tools to bring these methods to the general biomedical research community. These tools support the research of hundreds of thousands of investigators worldwide. Thus, to achieve its goals, the lab includes both computational biologists and software engineers working together to further our understanding and treatment of disease.
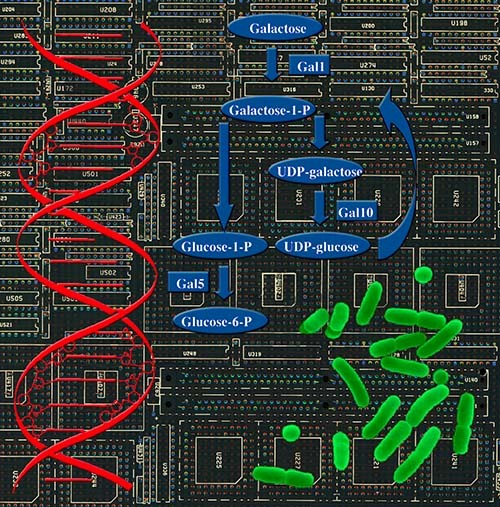
The work of the lab includes
- New statistical and machine learning techniques for cancer genomics. We develop and apply new machine learning and statistical techniques to find molecular markers to aid in the diagnosis, prognosis, and treatment of cancer as well as to understand the underlying biological processes. While we apply these methods to a wide range of tumor types, much of our work has focused on medulloblastoma, a pediatric brain tumor.
- New statistical and mathematical techniques for interpreting genomic data. From a biological perspective, we know it is not individual genes but rather sets of genes, representing pathways or states of the cell, that are the real features, by which we might predict the character of a particular patient sample. We made a major contribution in this area by the introduction of a statistical methodology called Gene Set Enrichment Analysis (GSEA), which is now standard practice for interpreting global transcription profiling data and has thousands of citations. This was the first of a number of signature-based approaches we developed and applied to study genomic data. More recently, we have introduced information theoretic approaches to help elucidate the genomic alterations associated with biological phenotypes and identify novel therapeutics to treat disease.
- Identifying states related to infectious disease. We studied the in vivo transcriptional profiles of the malaria parasite Plasmodium falciparum, i.e., from samples taken from the human hosts. Our computational methods enabled us to use signatures of known states in yeast (Saccharomyces cerevisiae) to identify previously unknown physiological diversity in P. falciparum. We used our signature-based approaches on pre-vaccination molecular profiles to identify vaccinees who will have a strong vs. weak immune response.
- Software and reproducible research: Our lab is dedicated to disseminating the mathematical and computational methods we develop as accessible software tools. These tools serve in aggregate over 300,000 investigators world-wide and include the GSEA software package and our comprehensive repository of gene sets, the Molecular Signatures Database (MSigDB) used with GSEA to interpret genomic data; an integrative computational genomics software environment, GenePattern; the Integrative Genomics Viewer (IGV), a high-performance visualization tool for interactive exploration of large, integrated genomic datasets; and the GenomeSpace platform which supports transparent interoperability between popular bioinformatics tools and data resources.
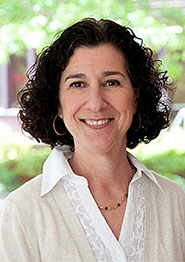
Jill P. Mesirov, Ph.D.
Professor of Medicine
Division of Medical Genetics;
Associate Vice Chancellor for Computational Health Sciences
UC San Diego School of Medicine
Moores Cancer Center UC San Diego
Locations
Dr. Mesirov's Research Office
Biomedical Research Facility Room 2A21
Vice Chancellor's Office, Biomedical Sciences Building Room 1309
UC San Diego La Jolla Campus, 9500 Gilman Drive Mail Code 0602, La Jolla, CA 92093